Silicon and Neuromorphic Photonics
Neuromorphic processors are widely considered one of the next frontiers in computing. We are fundamentally investigating information processing by leveraging the strengths of nanophotonic (i.e., optical) physics and neuromorphic (i.e., brain-inspired) architectures, and emerging technology (silicon and graphene photonics) platforms. We seek to develop programmable photonic processors that have the potential to outperform state-of-the-art and future microelectronic processors in energy efficiency and computational speeds by seven- and four orders of magnitude, respectively. Our research: 1) explores energy-efficient photonic neurons with graphene-based electro-optic modulators (devices theme); 2) develops ultrafast, scalable, photonic neural network architectures in silicon photonics platforms (architectures theme); and 3) advances the understanding of processors for generalized neuromorphic computing tasks including deep learning and nonlinear optimization (applications theme).
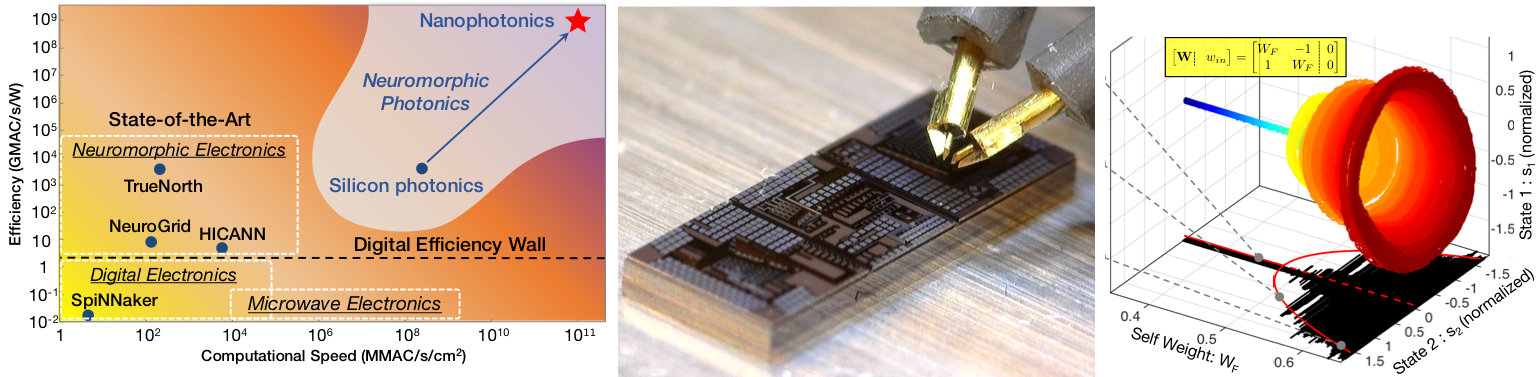
Quantum Neuromorphic Photonics
Quantum photonic neural networks (QPNNs) are brain-inspired, nonlinear photonic circuits that can learn to tackle many key challenges emerging quantum technologies face. By combining the strengths of artificial intelligence and photonic integrated circuits, these networks can learn to perform near-deterministic, high fidelity (i.e., near 100% chance of success) quantum processing as would be necessary for fundamental elements of the future quantum internet, for example. However, proposals of the network to date have assumed that all of its components work perfectly. Instead, we developed advanced simulations to model and analyze the performance of imperfect quantum photonic neural networks based on current state-of-the-art experimental capabilities, including the relevant imperfections. In doing so, we found that imperfect networks can still achieve near-deterministic operation and unraveled the intricate relationship between chip fabrication imperfections, weak optical nonlinearities, and network size, thus providing a guide to the optimal design of future quantum photonic neural networks for experimentally viable settings. Our results could enable the construction of viable, brain-inspired quantum photonic devices for emerging quantum technologies for quantum simulators, to process or reduce the noise of quantum states, and quantum machine learning.
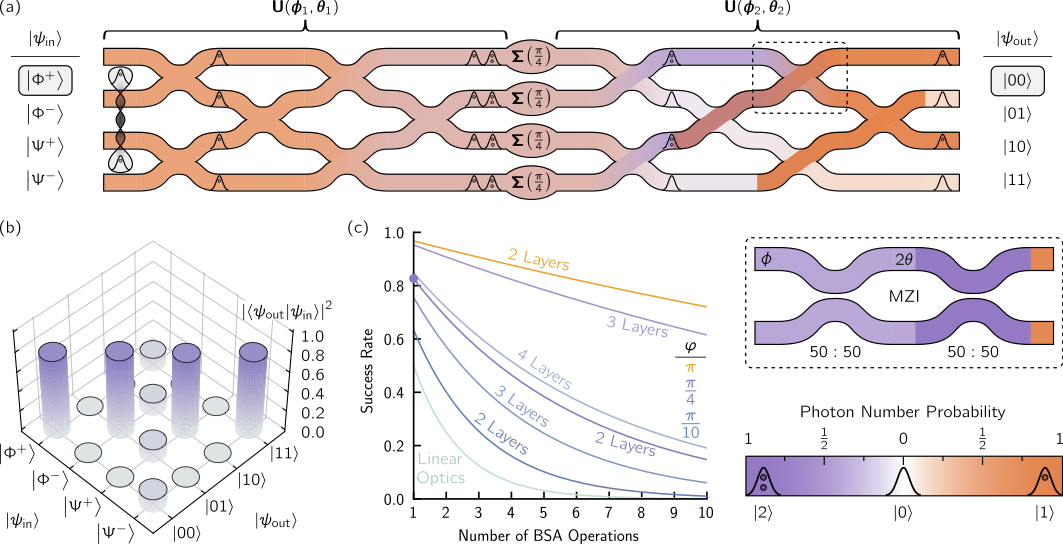
Laser Dynamics and Networks
We are studying laser dynamics and developing photonic integrated circuits in III-V and hybrid silicon photonics platforms with networks of lasers for spike-based information processing and chaos for optical secure communications. This paradigm takes advantage of ultrafast and low-energy phenomena in photonic devices, and has the potential for orders of magnitude performance improvement over current processing systems. Spike-based encoding scheme has both analog and digital qualities, and, via careful interleaving of the two representations, is potentially capable of achieving the high performance and low power characteristics of analog systems while simultaneously being cascadable and scalable. Our approach exploits both function-oriented models in optoelectronics and integrated interconnection strategies based on fiber optical networking.
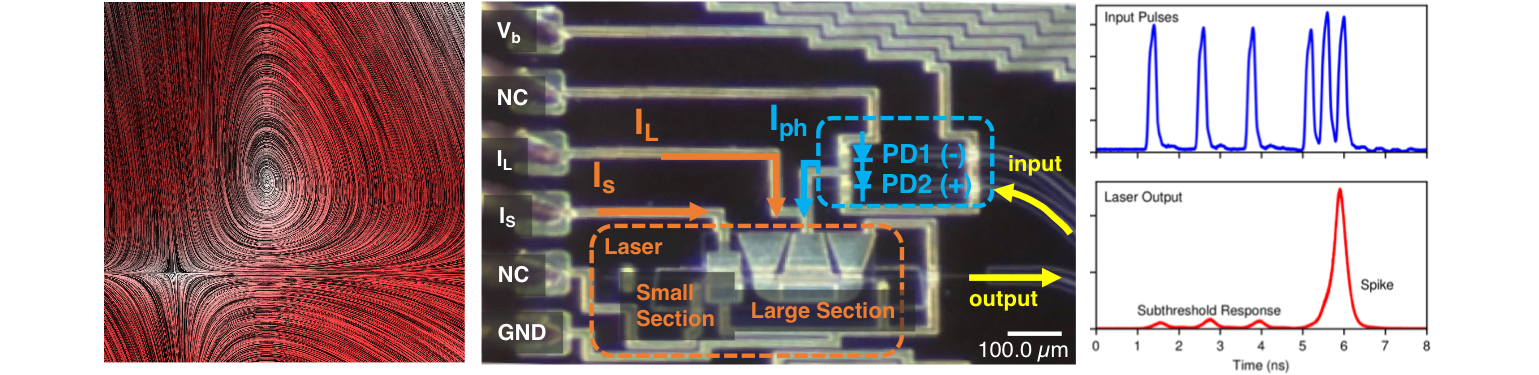