A new approach to critical care
June 29, 2022
Share
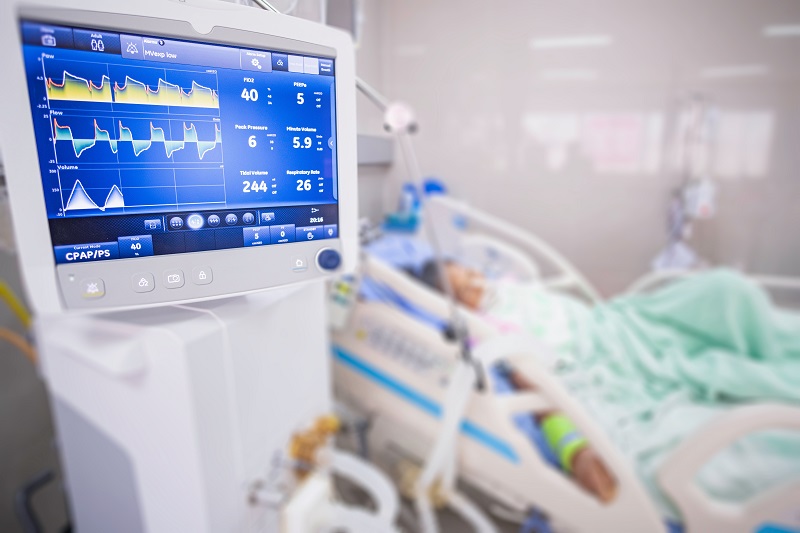
When patients are admitted to an intensive-care unit, health practitioners provide treatment based on the signs and symptoms they observe. This approach does not necessarily include understanding the definite causes behind those symptoms. However, even though some patients might present similar symptoms, the underlying biology of their illnesses can vary, which helps to explain why patients with the same signs and symptoms often respond differently to treatments.
In recent years, technological advances in molecular sciences, big data, and machine learning have allowed researchers to dig deeper in understanding illnesses and symptoms. This new knowledge can radically change how we approach critical care at the individual patient level.
In a recent paper published in Nature Medicine, David Maslove (School of Medicine) and colleagues argue it’s time for a paradigm shift in critical care. He spoke to the Gazette about how the COVID-19 pandemic advanced critical care research and what knowledge gaps we still need to address to put this new approach into practice.
How did critical care emerge as a discipline and why has it traditionally looked at syndromes to understand patients' needs and possible outcomes?
Critical care arose as a medical specialty in the mid-20th century, as the technology was advancing to provide organ support and assisted breathing. With that came a special set of skills and a unique environment within the hospital where the sickest patients could be closely monitored with specialized equipment and a strong nursing presence.
The use of syndromes to describe these cases is directly related. By syndrome, we mean a collection of signs and symptoms that together indicate to a provider that a specific condition is present. The syndromes are based on clinical manifestations – what the nurses and doctors observe. But they don't necessarily speak to what the underlying biology of the illness is.
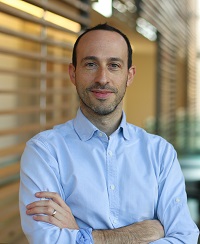
One example is sepsis, which is a syndrome of life-threatening organ dysfunction resulting from infection. Even though patients with sepsis have similar symptoms – fever, confusion, shortness of breath, high heart rate, and others – there are many different pathogens that can trigger the reaction, and a targeted treatment could be much more efficient than the current standards of care.
How is the advent of data science, machine learning, and molecular analyses affecting critical care and pushing it towards a new phase?
Over the last decade or two, as new tools in molecular medicine like genomics and gene expression profiling came into wider use, we began to apply them in critical care. Those technologies allow a much deeper look at what's going on at the level of the cell and the genome when somebody is critically ill. They also generate massive quantities of data – data that traditional statistical methods don't always handle in intuitive ways. That’s why machine learning approaches couple nicely with the new data that we are getting from these technologies: they reveal hidden patterns that can provide insights into the heterogeneity or diversity of critical illness syndromes.
What did critical care researchers and practitioners learn from COVID-19?
Because we had a group of critically ill patients that were all affected by the same pathogen – the COVID19 virus, SARS-CoV-2 – there was a tremendous opportunity to test potential treatments in a group that was more homogeneous than usual, increasing the chances that a therapy would on average affect everyone the same way. Researchers were able to promptly design and perform randomized clinical trials to test potential therapies, and they identified some effective treatments. Prior to that, because our clinical trials were treating heterogeneous groups, some patients benefitted from the treatment and others did not. It was harder to tell if the proposed treatment worked for anyone.
On a practical level, we learned some key lessons about the importance of research infrastructure to be able to collect and analyze data quickly, and the importance of being ready to efficiently enroll patients into clinical trials. We also reaffirmed that randomizing treatments is the best way to identify which ones work best. As critical care scientists from all over the world were working together towards a singular goal, we reaffirmed the importance of international collaboration.
What are the main gaps critical care research has yet to address?
We need to clarify what it means to respond to a treatment in the ICU context, where cases are so dynamic and complex that it can be difficult to understand whether a treatment has provided benefit in any given case.
There’s also a need for biomarkers that can be used to identify patients who are more or less likely to respond to a given treatment. In critical care, a particular challenge is that you can’t send a blood test off to a lab far away and wait for a complex testing like RNA sequencing to be completed. We need agile tests that can be done at the bedside.
How do you see future research changing the lives and outcomes of patients under critical care?
With this kind research, we are moving towards precision medicine, an approach pioneered in cancer treatment. The potential benefits are tremendous. By identifying which patients are most likely to respond to which treatments, we stand to increase the efficiency of the care that we provide. The precision medicine approach spares patients from ineffective treatments.
We know that everyone experiences critical illness differently and has different care needs, and we are looking at how to address those individual needs.